Introduction
Artificial Intelligence (AI) and Machine Learning (ML) are transforming the field of medical diagnostics, offering unprecedented accuracy and efficiency in detecting diseases early and tailoring treatments. This technological revolution is poised to significantly enhance patient outcomes and reduce healthcare costs.
The Role of AI and ML in Diagnostics
1. Early Disease Detection
AI and ML algorithms can analyze vast amounts of medical data to identify patterns and anomalies that might be indicative of early-stage diseases. Key areas of impact include:
- Cancer Detection: AI systems, such as those using deep learning, can detect early signs of cancers like breast, lung, and skin cancer from imaging data with higher accuracy than traditional methods.
- Cardiovascular Diseases: ML algorithms can predict the onset of heart diseases by analyzing ECGs, MRIs, and other diagnostic tests, often identifying risks invisible to the human eye.
2. Enhancing Medical Imaging
Medical imaging is a critical area where AI and ML are making substantial contributions:
- Radiology: AI-driven tools can analyze X-rays, CT scans, and MRIs more quickly and accurately, reducing the workload on radiologists and decreasing the chances of human error.
- Ophthalmology: AI systems can detect conditions such as diabetic retinopathy and macular degeneration from retinal scans, enabling earlier intervention and better patient outcomes.
3. Predictive Analytics and Risk Assessment
Predictive analytics powered by AI can assess patient data to predict disease risks and outcomes:
- Chronic Disease Management: AI models can analyze patient histories, lifestyle factors, and genetic data to predict the likelihood of developing chronic conditions like diabetes or hypertension.
- Hospital Readmissions: ML algorithms can identify patients at high risk of readmission, allowing healthcare providers to implement preventive measures.
Integration with Clinical Workflows
1. Decision Support Systems
AI-driven decision support systems assist healthcare professionals by providing data-driven insights:
- Diagnostic Assistance: These systems can suggest possible diagnoses based on patient symptoms and medical history, improving diagnostic accuracy.
- Treatment Recommendations: AI can recommend personalized treatment plans by analyzing data from similar patient cases and outcomes.
2. Workflow Automation
AI and ML streamline various administrative and clinical workflows:
- Electronic Health Records (EHR): AI can automate data entry and retrieval, reducing the time clinicians spend on paperwork and increasing time available for patient care.
- Appointment Scheduling: AI systems can optimize scheduling based on patient needs and resource availability, improving clinic efficiency.
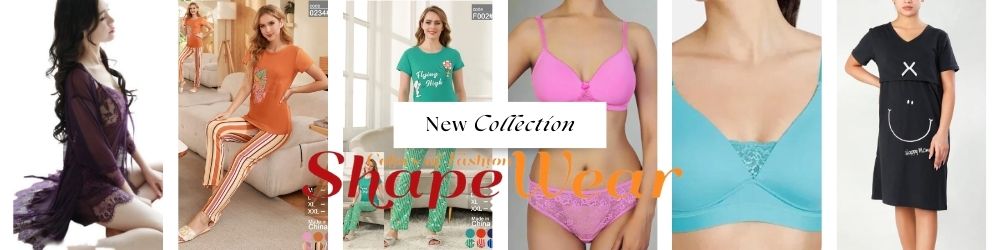
Challenges and Ethical Considerations
1. Data Privacy and Security
The use of AI in diagnostics involves handling vast amounts of sensitive patient data, raising concerns about privacy and security:
- Data Protection: Ensuring that patient data is securely stored and transmitted to prevent unauthorized access and breaches.
- Regulatory Compliance: Adhering to regulations like HIPAA (Health Insurance Portability and Accountability Act) to protect patient confidentiality.
2. Algorithm Bias and Fairness
AI systems can exhibit biases based on the data they are trained on, leading to unequal treatment outcomes:
- Bias Mitigation: Developing and implementing strategies to identify and eliminate biases in AI algorithms to ensure fair treatment across diverse patient populations.
- Transparency: Ensuring that AI decision-making processes are transparent and understandable to both clinicians and patients.
3. Integration with Existing Systems
Integrating AI and ML into existing healthcare infrastructure can be challenging:
- Compatibility: Ensuring that AI tools are compatible with current healthcare IT systems and workflows.
- Training and Adoption: Providing adequate training for healthcare professionals to effectively use AI tools and integrate them into their practice.
Future Prospects
1. Continuous Learning and Improvement
AI and ML systems continually learn and improve as they are exposed to more data:
- Adaptive Algorithms: Developing adaptive algorithms that can update and refine themselves based on new data, enhancing diagnostic accuracy over time.
- Collaborative Networks: Establishing networks where healthcare institutions can share data and insights, fostering collective learning and advancements in AI diagnostics.
2. Global Health Impact
AI and ML have the potential to improve healthcare access and quality globally:
- Resource-Limited Settings: AI tools can provide diagnostic support in resource-limited settings where specialist healthcare providers are scarce.
- Telemedicine: Integrating AI with telemedicine platforms can extend diagnostic capabilities to remote and underserved areas, improving healthcare accessibility.
Conclusion
AI and ML are revolutionizing medical diagnostics, offering the potential for earlier disease detection, enhanced imaging analysis, predictive analytics, and streamlined clinical workflows. Despite challenges related to data privacy, algorithm bias, and integration, the future of AI in diagnostics looks promising. Continued advancements in AI technology and collaborative efforts among healthcare stakeholders will further enhance diagnostic accuracy and patient outcomes, ultimately transforming the landscape of modern medicine.