Introduction
Artificial Intelligence (AI) and Machine Learning (ML) are revolutionizing the field of medical sciences, transforming how healthcare is delivered, diagnoses are made, and treatments are personalized. This paper explores the extensive applications, current trends, ethical considerations, challenges, and future prospects of AI and ML in medical sciences.
Historical Background
The journey of AI in medicine began in the mid-20th century, with early attempts to create expert systems that could mimic human reasoning. However, it was not until the advent of more advanced computing power and the development of sophisticated algorithms in the 21st century that AI and ML began to make significant inroads into medical sciences.
Core Concepts in AI and ML
- Artificial Intelligence (AI):
- Definition: AI refers to the simulation of human intelligence in machines designed to think and act like humans. In medical sciences, AI encompasses a wide range of applications, from simple automation to complex decision-making systems.
- Types: Narrow AI, which performs specific tasks, and General AI, which possesses generalized human cognitive abilities (still theoretical).
- Machine Learning (ML):
- Definition: ML is a subset of AI that involves training algorithms on large datasets to learn patterns and make predictions or decisions without being explicitly programmed for specific tasks.
- Types: Supervised learning, unsupervised learning, semi-supervised learning, and reinforcement learning.
- Deep Learning:
- Definition: A subset of ML that uses neural networks with many layers (deep neural networks) to model complex patterns in data. It is particularly effective in image and speech recognition tasks.
- Applications: Deep learning has become a cornerstone in medical image analysis, natural language processing (NLP), and genomics.
Applications of AI and ML in Medical Sciences
- Medical Imaging and Diagnostics:
- Radiology: AI algorithms, especially convolutional neural networks (CNNs), are used to analyze medical images (X-rays, MRIs, CT scans) for detecting abnormalities such as tumors, fractures, and lesions. For example, Google’s DeepMind developed an AI system capable of diagnosing eye diseases from retinal scans with accuracy comparable to human experts.
- Pathology: AI assists in analyzing histopathological images to identify cancerous cells, reducing the workload of pathologists and increasing diagnostic accuracy. Whole-slide imaging combined with AI can detect subtle histological changes indicative of disease.
- Predictive Analytics:
- Risk Prediction: ML models are used to predict patient outcomes, such as the likelihood of developing certain diseases or the probability of readmission after surgery. These models analyze vast amounts of patient data, including medical history, genetic information, and lifestyle factors, to identify at-risk individuals.
- Sepsis Detection: AI systems can monitor patient vitals in real-time and alert healthcare providers to early signs of sepsis, a life-threatening condition, enabling timely intervention.
- Personalized Medicine:
- Genomics: AI and ML are instrumental in analyzing genomic data to identify genetic mutations associated with diseases. This enables the development of personalized treatment plans based on an individual’s genetic makeup.
- Drug Discovery: AI accelerates drug discovery by predicting how different compounds will interact with biological targets. Companies like Atomwise and Insilico Medicine use AI to screen millions of molecules for potential drug candidates, significantly reducing the time and cost of drug development.
- Natural Language Processing (NLP):
- Electronic Health Records (EHRs): NLP algorithms can extract meaningful information from unstructured data in EHRs, such as clinical notes and medical literature. This helps in clinical decision support, identifying patient cohorts for research, and ensuring accurate coding for billing purposes.
- Virtual Assistants: AI-powered virtual assistants like IBM’s Watson are used to support clinical decision-making by providing evidence-based recommendations based on vast medical databases.
- Robotics and Surgery:
- Robotic Surgery: AI enhances robotic surgery systems, enabling greater precision and control. For example, the da Vinci Surgical System uses AI to assist surgeons in performing minimally invasive procedures with high accuracy.
- Rehabilitation Robotics: AI-driven robots are used in rehabilitation to assist patients in recovering motor functions. These robots adapt to the patient’s progress and provide personalized therapy sessions.
- Telemedicine and Remote Monitoring:
- Telehealth: AI-powered telemedicine platforms facilitate remote consultations and monitoring, especially important in rural and underserved areas. These platforms use AI to triage patients, recommend treatments, and monitor chronic conditions.
- Wearables: AI algorithms analyze data from wearable devices to monitor patient health in real-time, providing insights into physical activity, heart rate, sleep patterns, and other vital signs.
Current Trends in AI and ML in Medical Sciences
- Explainable AI (XAI):
- Transparency: There is a growing emphasis on developing AI systems that can explain their decision-making process. This transparency is crucial for gaining trust from healthcare providers and patients.
- Interpretability: Techniques such as feature importance, LIME (Local Interpretable Model-agnostic Explanations), and SHAP (SHapley Additive exPlanations) are used to interpret complex AI models.
- Federated Learning:
- Privacy Preservation: Federated learning enables training ML models across multiple institutions without sharing sensitive patient data. This collaborative approach enhances model performance while maintaining data privacy.
- Data Integration: Combining data from various sources (hospitals, clinics, research institutions) leads to more robust and generalizable AI models.
- AI in Pandemic Response:
- COVID-19: During the COVID-19 pandemic, AI was used for various purposes, including predicting disease spread, identifying high-risk patients, accelerating vaccine development, and analyzing CT scans for infection severity.
- Epidemiology: AI continues to play a vital role in monitoring and managing public health crises by analyzing trends, predicting outbreaks, and optimizing resource allocation.
Ethical Considerations and Challenges
- Bias and Fairness:
- Algorithmic Bias: AI systems can perpetuate or even exacerbate existing biases in healthcare if trained on unrepresentative or biased data. Ensuring diversity in training datasets and implementing fairness-aware algorithms are crucial steps.
- Equity in Healthcare: AI should be used to promote health equity, ensuring that all patient groups benefit equally from technological advancements.
- Privacy and Security:
- Data Privacy: Protecting patient data is paramount. Compliance with regulations such as GDPR (General Data Protection Regulation) and HIPAA (Health Insurance Portability and Accountability Act) is essential for maintaining trust and legal standards.
- Cybersecurity: As healthcare systems become more interconnected, robust cybersecurity measures are necessary to protect against data breaches and cyberattacks.
- Regulation and Standards:
- Regulatory Frameworks: Developing clear and comprehensive regulatory frameworks for AI in healthcare is necessary to ensure safety, efficacy, and accountability. Regulatory bodies like the FDA (Food and Drug Administration) are working on guidelines for AI-based medical devices and software.
- Standardization: Establishing standards for data formats, model validation, and performance metrics is essential for the interoperability and reproducibility of AI systems.
- Integration into Clinical Practice:
- Workflow Integration: Successfully integrating AI into clinical workflows requires seamless integration with existing systems, user-friendly interfaces, and adequate training for healthcare providers.
- Adoption Barriers: Resistance to change, lack of trust in AI systems, and concerns about job displacement are potential barriers to adoption that need to be addressed through education and demonstration of AI’s value.
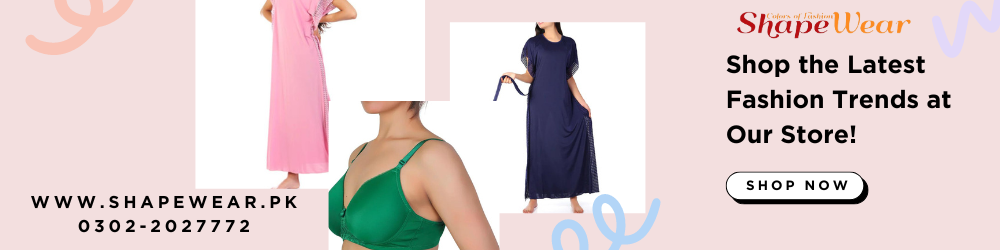
Future Prospects of AI and ML in Medical Sciences
- AI-Driven Drug Development:
- Accelerated Discovery: AI is expected to significantly shorten the drug development timeline by identifying potential drug candidates, predicting clinical trial outcomes, and personalizing treatment regimens.
- Precision Medicine: Integration of genomic, proteomic, and clinical data through AI will lead to highly personalized treatment plans, optimizing therapeutic efficacy and minimizing adverse effects.
- AI and Robotics in Surgery:
- Autonomous Surgical Systems: The development of fully autonomous surgical robots is a potential future direction, with AI guiding real-time decision-making and execution of complex surgical procedures.
- Enhanced Capabilities: AI will continue to enhance the capabilities of robotic systems, improving surgical precision, reducing recovery times, and increasing the success rates of complex surgeries.
- AI in Mental Health:
- Early Detection and Intervention: AI can analyze patterns in speech, social media activity, and biometric data to detect early signs of mental health issues and provide timely interventions.
- Personalized Therapy: AI-powered chatbots and virtual therapists are being developed to offer personalized mental health support, augmenting traditional therapy methods.
- Global Health and AI:
- Resource Optimization: AI can help optimize resource allocation in low-resource settings, improving access to healthcare and quality of care in underserved regions.
- Disease Surveillance: AI-powered systems for real-time disease surveillance and outbreak prediction will enhance global health security and response capabilities.
- Continuous Learning Systems:
- Adaptive Algorithms: AI systems that continuously learn and adapt based on new data and feedback will improve over time, leading to more accurate and reliable healthcare solutions.
- Collaborative AI: Collaboration between AI systems and human experts will lead to better outcomes, leveraging the strengths of both to address complex medical challenges.
Conclusion
AI and ML are poised to transform medical sciences, offering unprecedented opportunities to enhance diagnosis, treatment, and patient care. While the potential benefits are immense, addressing ethical considerations, ensuring data privacy, and overcoming integration challenges are essential for realizing the full potential of AI in healthcare. As the field continues to evolve, collaborative efforts among